How to Become a Full-Stack Data Scientist Unicorn
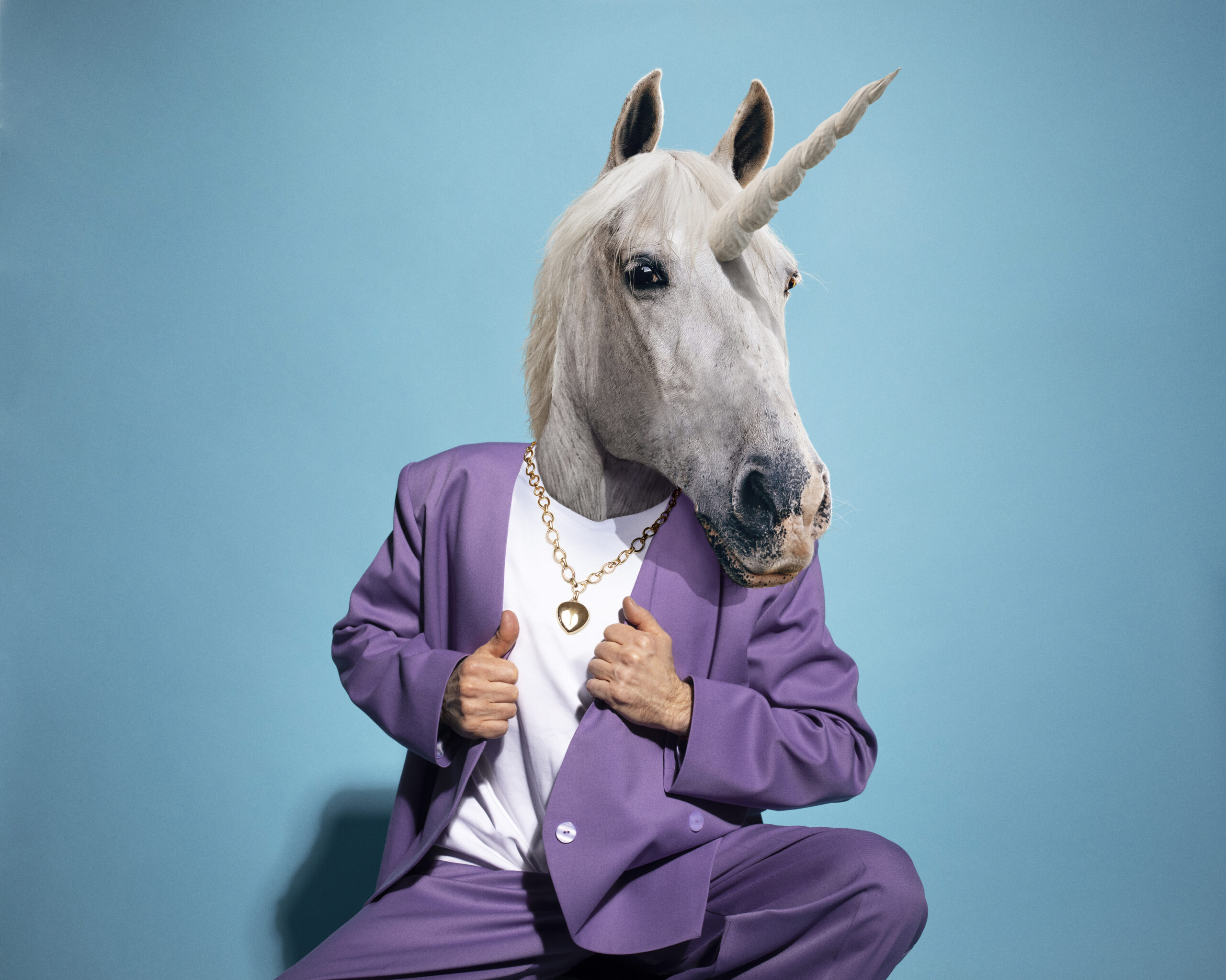
A few years ago, I hired one of my best data scientists. Here’s the gist of how the interview went.
- Me: How well do you know Unix?
- Candidate: I run a Raspberry Pi cluster at home using Unix. Would that count?
- Me: You’re hired!
This data scientist had a marketing degree, yet was hands-on in setting up Raspberry Pi computers as a hobby. I knew I found the mythical “unicorn” in the form of a full-stack data scientist.
I hustled to hire him before other data science managers realized his potential. He ended up being a stellar hire for the company that everyone else later also wanted on their teams. As his manager, I was able to promote him to staff in a year, a rare feat.
What makes a full-stack data scientist so attractive to employers?
Which Career Path to Take?
Like many other roles, every data scientist faces a career choice at some point. Which career path to take?
- Aim to become a people manager and eventually a general manager?
- Aim to become an IC senior staff, principal, or fellow deep in one data science or AI sub-specialty?
- Aim to become a full-stack data scientist in a startup?
- Or leave data science entirely to become a product manager in a big company?
For each of the above paths, I know different data scientists who have chosen to take each of them. There’s no one right or wrong answer for everyone.
Which path would you choose if you’re currently in data science?
You would certainly weigh your abilities, interests, and market potential before deciding.
- Do you like leading and influencing people?
- Do you like doing research and publishing and teaching?
- Do you like hacking together an app from end-to-end?
- Do you like prioritizing resources for building meaningful solutions?
If you don’t make a career plan for yourself, then someone else, like your employer, may place a generic career plan for you that doesn’t fit you.
The Full-Stack Data Scientist
For today, I want to explore the role and career path of the full-stack data scientist with you.
A full-stack data scientist is someone who can deftly handle the entire data science workflow from end-to-end, single-handedly.
As a data engineer, the full-stack data scientist knows how to:
- Collect data (scrape or use APIs as needed)
- Parse, wrangle, and process the data (knows ETL, ELT, SCD, surrogate keys, etc.)
- Make the data reliable, secure, and cost effective (uses cloud services with SSH tunnels)
As a business data analyst, the full-stack data scientist knows how to:
- Understand the business domain and business metrics
- Present findings and recommendations to senior leaders
- Apply statistical rigor to analyses, experiments, and business metrics
As an AI/ML expert, the full-stack data scientist knows how to:
- Build supervised and unsupervised learning models
- Design and tune hyper-parameters of an ML model
- Run TensorFlow and PyTorch and other massively parallel deep learning frameworks
As an app builder, the full-stack data scientist hows how to:
- Build web apps (both front-end and back-end)
- Build web browser extensions (e.g. Chrome “plugin”)
- Build data visualizations, not just from tools, but also from code
The full list above sounds like such high expectations. How can anyone learn to do all these things?
Do these “unicorns” really exist?
Well, the full-stack data scientist that I hired a few years ago was able to do each of the above bullet points. Plus, he found and fixed a bug in the source code of the NumPy Python library that’s core to what virtually every data scientist uses today. He earned our deep respect.
I would consider a full-stack data scientist to be a “10x” data scientist, supposedly as versatile and productive as the sum of 10 typical data scientists.
I’ve seen such full-stack data scientists rise up from data scientists who run hobby data projects for fun outside of their day jobs. They have a DIY mindset, just like my example data scientist who built a Raspberry Pi cluster at home for fun.
Beyond technical abilities, these full-stack data scientists also excel in communications and business too. As a result, I know full-stack data scientists who have become founders of startups.
In a sense, these full-stack data scientists are the ultimate generalists. Yet, they still seem to excel in every step needed in the workflow of building data solutions.
Ultimately, as big enterprises tend to want deep specialists to fulfill narrow job descriptions, these full-stack data scientists yearn for a better fit in the startup world.
What is Your Best Fit Career Path?
Back to my original question, which career path would you want to take as a data scientist?
If you like building apps end-to-end, even as a hobby, then you may already be a full-stack data scientist. Just be aware that full-stack data scientists, with their inherent 10x productivity, may find a better fit in a startup than in a big enterprise.
You don’t need to be a full-stack data scientist unicorn in order to have a joyful career though.
You may want to become a people manager, a product manager, or a high-level individual contributor data scientist for your ideal career path. All these paths are viable paths for data scientists to succeed. You just need to pick the path that personally fits you the best.
As a former data science senior manager from LinkedIn, I now coach data professionals to thrive in the age of AI. Contact me here or via social media to learn more.
Feature image by freepik, used with permission
Originally sent via Jimmy Wong’s weekly newsletter: Full-Stack Data Scientist Unicorns
Subscribe for more tips:
We respect your privacy. Unsubscribe at any time.